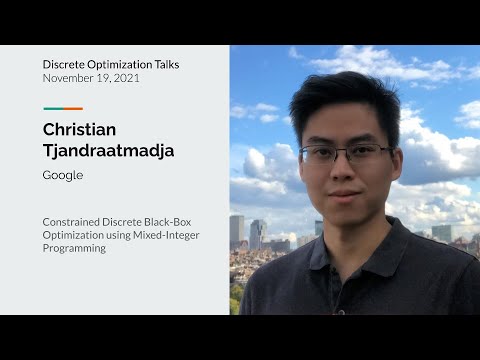
Part of Discrete Optimization Talks: https://talks.discreteopt.com
Christian Tjandraatmadja - Google
Constrained Discrete Black-Box Optimization using Mixed-Integer Programming
Speaker webpage: https://research.google/people/ChristianTjandraatmadja/
Abstract:
Discrete black-box optimization problems are challenging for model-based optimization (MBO) algorithms, such as Bayesian optimization, due to the size of the search space and the need to satisfy combinatorial constraints. In particular, these methods require repeatedly solving a complex discrete global optimization problem in the inner loop, where popular heuristic inner-loop solvers introduce approximations and are difficult to adapt to combinatorial constraints. In response, we propose NN+MILP, a general discrete MBO framework using piecewise-linear neural networks as surrogate models and mixed-integer linear programming (MILP) to optimize the acquisition function. MILP provides optimality guarantees and a versatile declarative language for domain-specific constraints. We test our approach on a range of unconstrained and constrained problems, including DNA binding and the NAS-Bench-101 neural architecture search benchmark. NN+MILP surpasses or matches the performance of algorithms tailored to the domain at hand, with global optimization of the acquisition problem running in a few minutes using only standard software packages and hardware.
This talk is based on the paper at https://arxiv.org/abs/2110.09569.
Bio:
Christian Tjandraatmadja is a software engineer in the Operations Research team at Google Research. His current research interests revolve around the intersection between mixed-integer programming and neural networks. He has a PhD in Algorithms, Combinatorics, and Optimization from the Tepper School of Business at Carnegie Mellon University.
0 Comments